Hello! I’m Shangzhe Li, an undergraduate student at South China University of Technology and an incoming Ph.D. student at UNC Chapel Hill, where I will be fortunate to work under the guidance of Prof. Weitong Zhang. I also collaborate closely with Prof. Hao Su at the University of California, San Diego. Previously, I’ve had the opportunity to work with Prof. Marco Caccamo and Prof. Nils Thuerey at the Technical University of Munich, as well as with Prof. Xinhua Zhang at the University of Illinois at Chicago.
I’m passionate about aviation, Physics, and Mathematics, and I’m pursuing my studies in Artificial Intelligence. My hometown is Guangzhou. In my free time, I enjoy live streaming on Bilibili, sharing insights on Zhihu, and indulging in my love for anime.
My research interests lie broadly in the fields of reinforcement learning and imitation learning, with a focus on both algorithmic design and practical applications:
-
Deep Reinforcement Learning: I’m interested in developing novel reinforcement learning algorithms powered by deep neural networks to tackle complex decision-making problems. Lately, I’ve been particularly focused on model-based RL.
-
Imitation Learning: I explore new approaches to imitation learning, especially adversarial imitation learning in complex, high-dimensional environments. I’m also excited about leveraging large foundation models within imitation learning frameworks.
-
Theoretical Foundations of RL/IL: I aim to better understand the theoretical underpinnings of reinforcement and imitation learning, with the goal of using theory to inform and inspire more effective algorithmic designs.
-
Applications in LLM Post-training and Robot Learning: I’m interested in applying RL to enhance large language model post-training (e.g., alignment, distillation, and reasoning), as well as to solve challenging robotic control problems in manipulation and locomotion.
Links to my social media:
🔥 News
- 2025.05, One paper has been accepted by ICML 2025.
- 2025.03, I’ll be joining UNC Chapel Hill for my PhD, advised by Prof. Weitong Zhang!
- 2025.03, One paper has been accepted by ICLR 2025 Workshop on World Models.
- 2024.03, Summer intern offer received from Su Lab, UCSD! See you in San Diego in summer if everything goes smoothly!
- 2023.09, 🎉🎉 Homepage has been set up.
📝 Publications
†: Equal contributions.
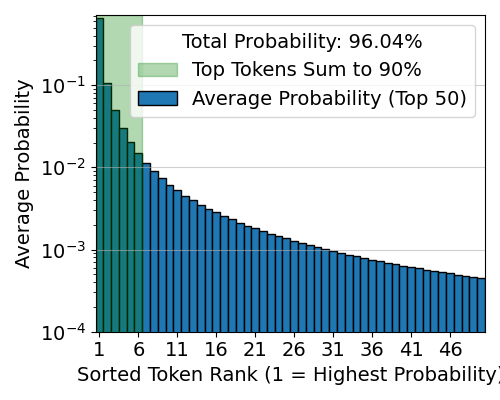
Language Model Distillation: A Temporal Difference Imitation Learning Perspective
Zishun Yu †, Shangzhe Li †, Xinhua Zhang
- Motivated by the observation that a small number of top tokens contribute the majority of the total probability mass, we proposed a top-p TD learning framework that operates over a top-p candidate action subset. This framework is, in principle, plug-and-play and can be integrated into any TD-based IRL algorithm. We demonstrated its practicality through a concrete implementation of top-p IQL and showed its empirical performance gains.
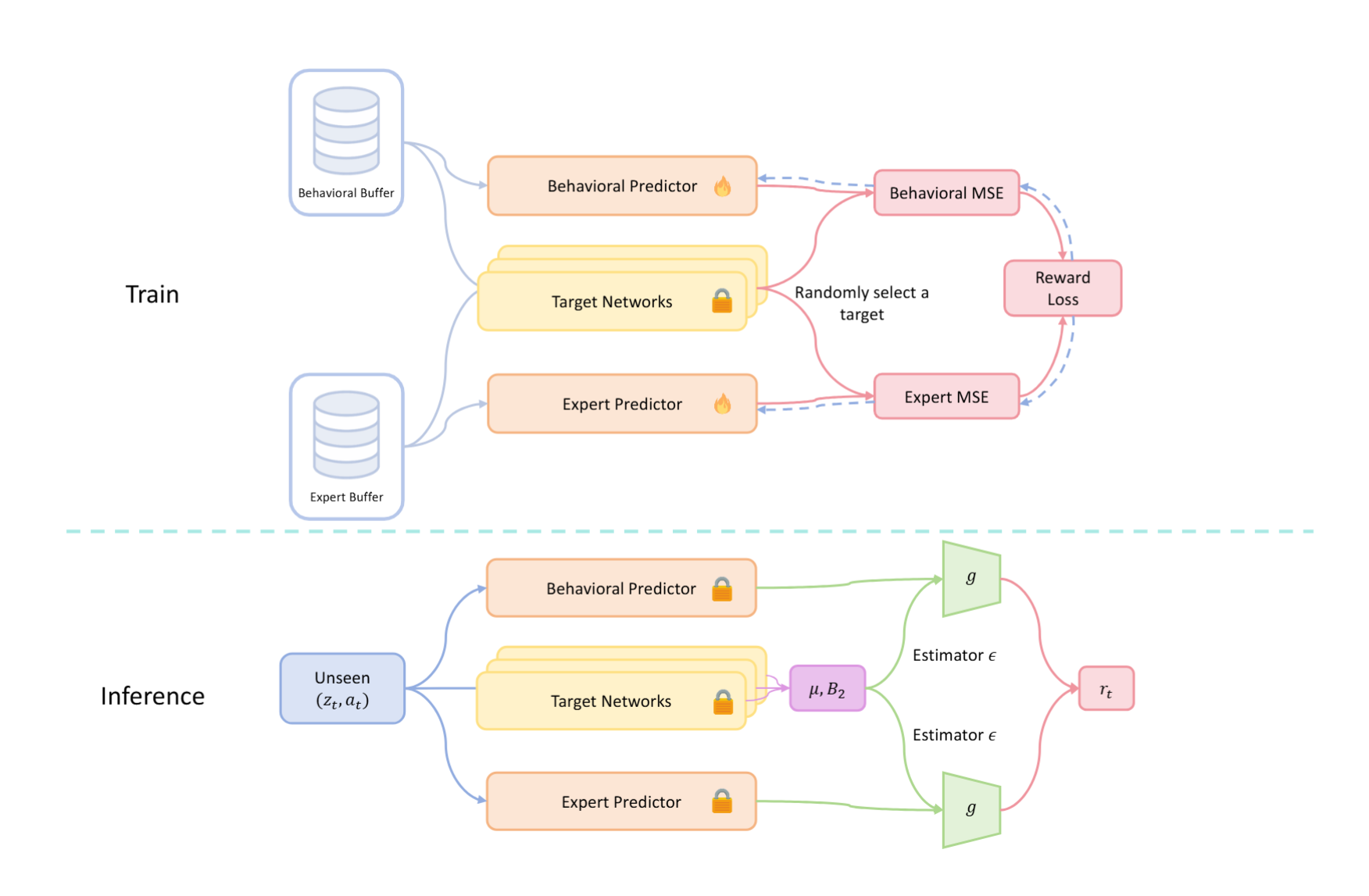
Coupled Distributional Random Expert Distillation for World Model Online Imitation Learning
Shangzhe Li, Zhiao Huang, Hao Su
- We propose a novel approach for world model-based online imitation learning, featuring an innovative reward model formulation. Unlike traditional adversarial approaches that may introduce instability during training, our reward model is grounded in density estimation for both expert and behavioral state-action distributions. This formulation enhances stability while maintaining high performance. Our model demonstrates expert-level proficiency across various tasks in multiple benchmarks, including DMControl, Meta-World, and ManiSkill2. Furthermore, it consistently retains stable performance throughout long-term online training. With its robust reward modeling and stability, our approach has the potential to tackle complex real-world robotics control tasks, where reliability and adaptability are crucial.
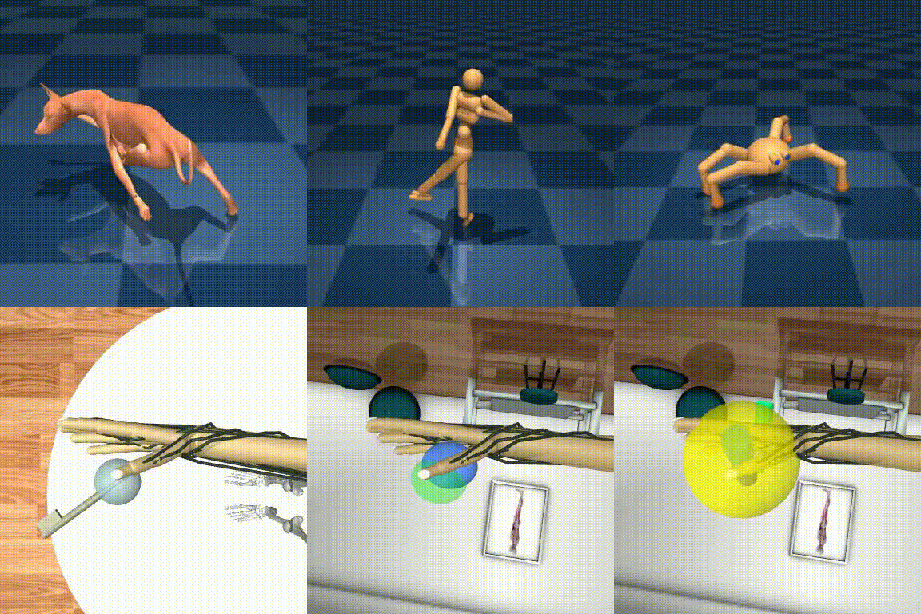
Reward-free World Models for Online Imitation Learning
Shangzhe Li, Zhiao Huang, Hao Su
- We propose an online imitation learning approach that utilizes reward-free world models to address tasks in complex environments. By incorporating latent planning and dynamics learning, our model can have a deeper understanding of intricate environment dynamics. We demonstrate stable, expert-level performance on challenging tasks, including dexterous hand manipulation and high-dimensional locomotion control.
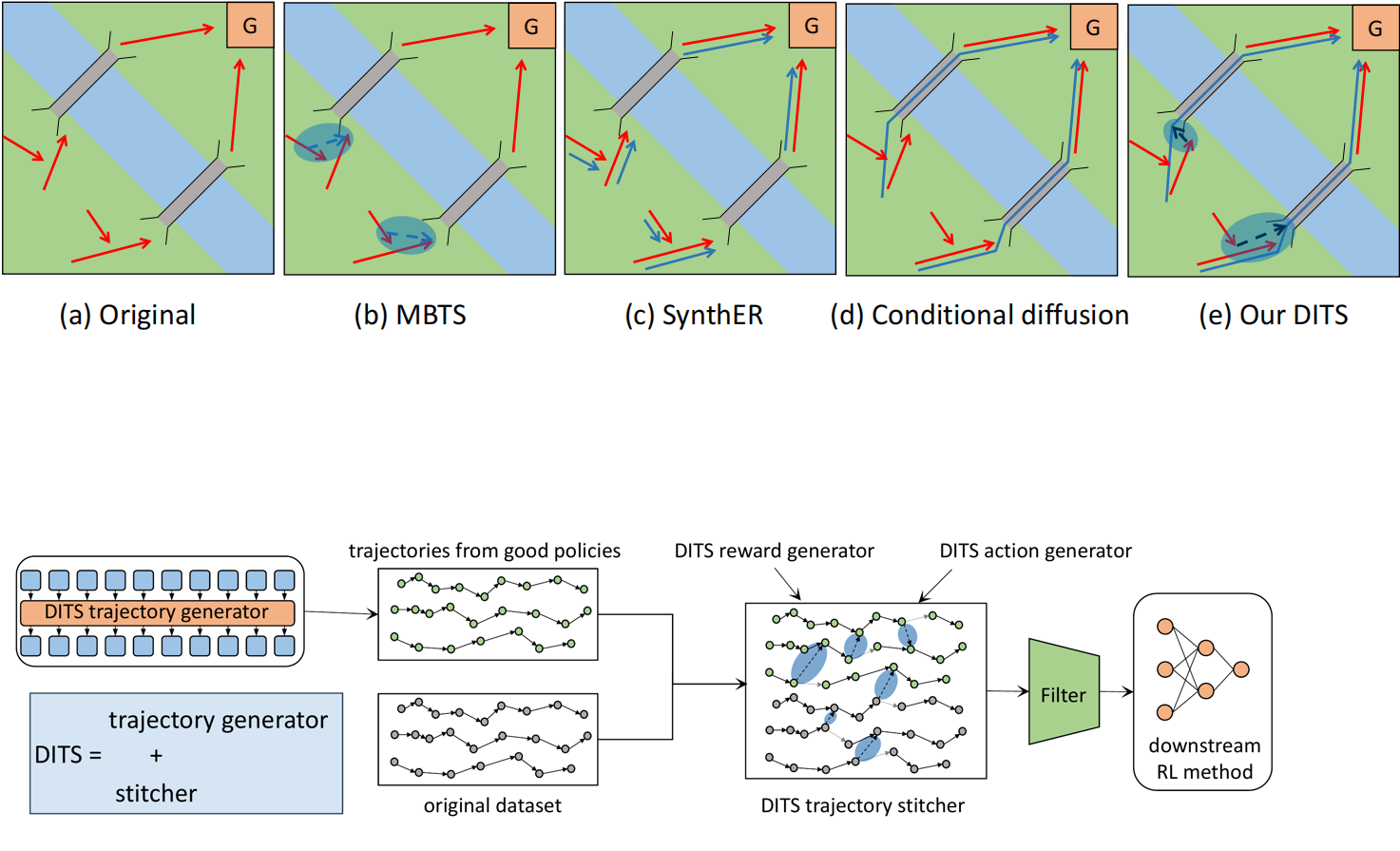
Augmenting Offline Reinforcement Learning with State-only Interactions
Shangzhe Li, Xinhua Zhang
- We proposed a novel data augmentation method DITS for offline RL, where state-only interactions are available with the environment. The generator based on conditional diffusion models allows high-return trajectories to be sampled, and the stitching algorithm blends them with the original ones. The resulting augmented dataset is shown to significantly boost the performance of base RL methods.
🎖 Honors and Awards
- 2022 First Prize, Asia and Pacific Mathematical Contest in Modeling(APMCM)
- 2022 Second Prize, National Contemporary Undergraduate Mathematical Contest in Modeling(CUMCM)
- 2022 Successful Participant, Mathematical Contest in Modeling(MCM)
- 2023 Successful Participant, Mathematical Contest in Modeling(MCM)
- 2021 Second Prize, Baidu “Paddle Paddle” Cup
- 2022 First Prize, Taihu Academic Innovation Scholarship (CNY 8000)
- 2022 Second Prize, Taihu Science Innovation Scholarship (CNY 5000)
📖 Educations
- 2023.10 - 2024.07, Exchange student, Technical University of Munich.
- 2021.09 - now, Undergraduate student, South China University of Technology.
- 2018.09 - 2021.06, High school student (Physics Olympiad), Affiliated High School of South China Normal University.
💻 Internships and Research Experience
- 2022.09 - 2023.04, Undergraduate Research, Neural Networks Compression and Acceleration Research. Supervisor: Prof. Ye Liu, South China University of Technology.
- 2023.05 - 2024.01, Research Intern (Remote), Application of Diffusion Model on Offline Reinforcement Learning. Supervisor: Prof. Xinhua Zhang, University of Illinois Chicago.
- 2023.10 - 2024.06, Research Intern, Research on the Control Approach for Two-way Coupled Fluid Simulation. Supervisor: Prof. Nils Thuerey, Technical University of Munich, Mentor: Patrick Schnell.
- 2024.01 - 2024.09, Research Intern, Research on the Fast Adaptation Methods on Reinforcement Learning. Supervisor: Prof. Marco Caccamo, Technical University of Munich, Collaborator: Hongpeng Cao.
- 2024.02 - now, Research Intern (Remote), Distillation in LLMs via Reinforcement Learning Algorithms. Supervisor: Prof. Xinhua Zhang, University of Illinois Chicago, Collaborator: Zishun Yu.
- 2024.06 - now, Research Intern, Research in World Models and Inverse Reinforcement Learning. Supervisor: Prof. Hao Su, University of California, San Diego, Mentor: Zhiao Huang.
📝 Blog Articles
Notice: All of the articles here are written in Chinese.
Physics Part:
- Propagation of electromagnetic waves in the presence of conductors
- Solution of Laplace’s equation in spherical coordinate system
- Gauge transformations and D’Alembert’s equation
- Maxwell stress tensor and electromagnetic field momentum
- Electrodynamics of tensor forms
- Geodesic equations, affine connections and covariant differentiation
- Intrinsic time variations, geodesic equations advanced
- Riemann curvature tensor
- Curvature advanced and Bianchi Constant Equation
- Energy-Momentum tensor and Einstein field equations
- Relativistic electrodynamics and electromagnetic analogies
- Harmonic coordinate condition
- Energy, momentum and angular momentum of a gravitational field
- Schwarzschild metric and Schwarzschild black hole
- Gravitational wave
- Gravitational wave advanced
- Universal definition of the action and energy tensor
- Energy-Momentum tensor advanced and gravitational action
- Killing vector fields and maximal symmetric spaces
- Lie group and Lie algebra
- Quantum Lorentz transformations and Poincaré algebra
- Single particle state
- Parity and time reversal
Mathematics Part:
- Dual spaces and tensors
- Symmetry of the tensor
- Continuous and discrete time Fourier transforms
- Laplace transform and Z-transform
- Primary fiber bundles (I)
- Primary fiber bundles (II)
- Connections on primary fibre bundles
Convex Optimization Part: